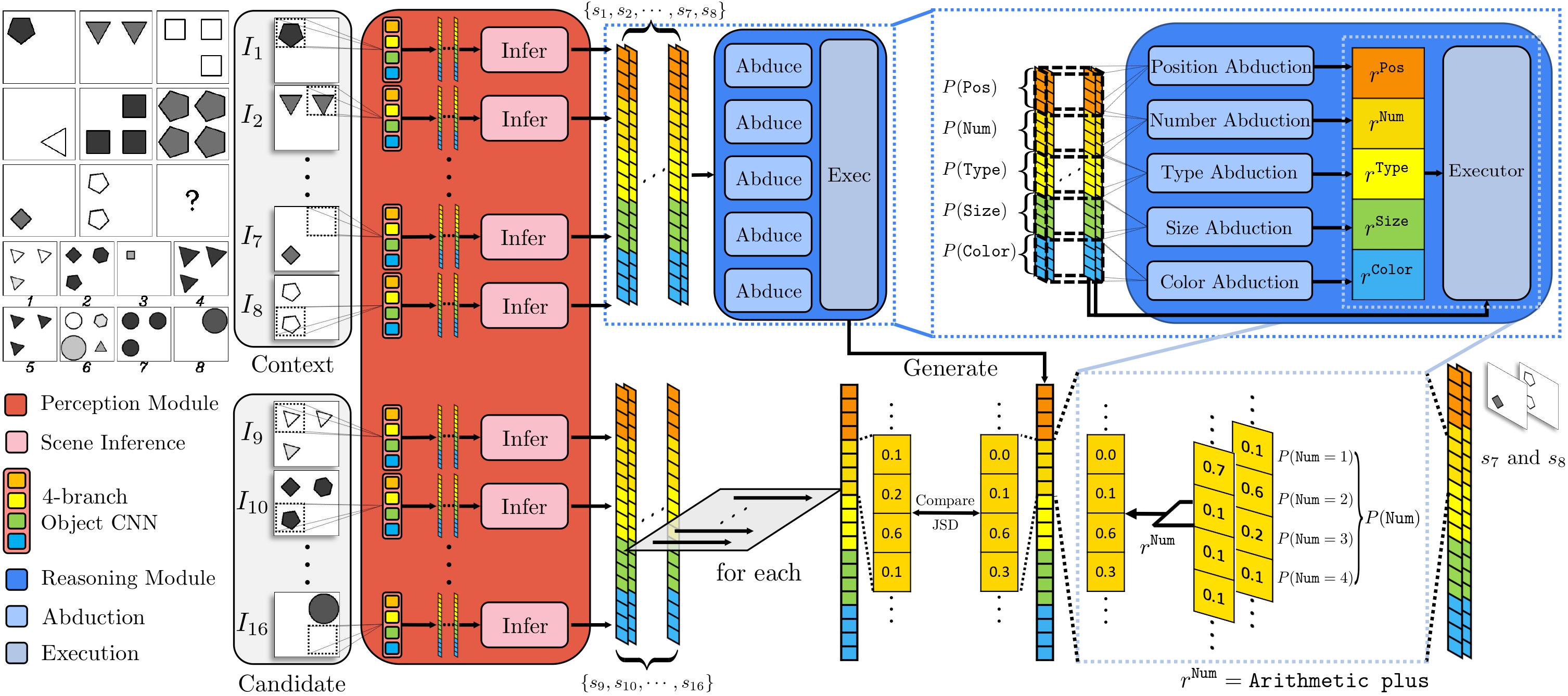
Spatial-temporal reasoning is a challenging task in Artificial Intelligence (AI) due to its demanding but unique nature: a theoretic requirement on representing and reasoning based on spatial-temporal knowledge in mind, and an applied requirement on a high-level cognitive system capable of navigating and acting in space and time. Recent works have focused on an abstract reasoning task of this kind -- Raven’s Progressive Matrices (RPM). Despite the encouraging progress on RPM that achieves human-level performance in terms of accuracy, modern approaches have neither a treatment of human-like reasoning on generalization, nor a potential to generate answers. To fill in this gap, we propose a neuro-symbolic Probabilistic Abduction and Execution (PrAE) learner; central to the PrAE learner is the process of probabilistic abduction and execution on a probabilistic scene representation, akin to the mental manipulation of objects. Specifically, we disentangle perception and reasoning from a monolithic model. The neural visual perception frontend predicts objects' attributes, later aggregated by a scene inference engine to produce a probabilistic scene representation. In the symbolic logical reasoning backend, the PrAE learner uses the representation to abduce the hidden rules. An answer is predicted by executing the rules on the probabilistic representation. The entire system is trained end-to-end in an analysis-by-synthesis manner without any visual attribute annotations. Extensive experiments demonstrate that the PrAE learner improves cross-configuration generalization and is capable of rendering an answer, in contrast to prior works that merely make a categorical choice from candidates.